Optimize dual frame¶
This document shows you how to optimize the post-processing of the POVM samples to improve expectation value estimations.
[1]:
%load_ext autoreload
%autoreload 2
Getting the POVM samples¶
Define quantum circuit¶
[2]:
from qiskit.circuit.random import random_circuit
qc = random_circuit(5, 3, measure=False, seed=875)
qc.draw("mpl", style="iqp")
[2]:
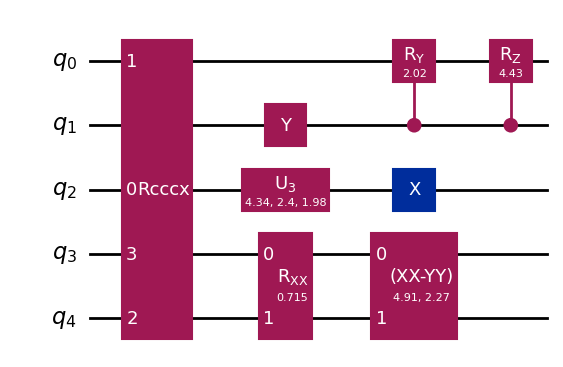
Define measurement procedure¶
[3]:
import numpy as np
from numpy.random import default_rng
from povm_toolbox.library import RandomizedProjectiveMeasurements
rng = default_rng(96568)
num_qubits = qc.num_qubits
bias = np.array([0.5, 0.25, 0.25])
angles = np.array(
[
[2.01757238, -1.85001671, 2.52155716, 0.45636669, 1.17175533, -0.48263278],
[0.0, 0.0, 1.57079633, -2.35619449, 1.57079633, -0.78539816],
[1.94493547, -2.39620342, 0.3760775, -2.28966468, 1.53443501, 2.33046898],
[0.0, 0.0, 1.57079633, 0.6, 1.57079633, 2.17079633],
[0.0, 0.0, 1.57079633, 0.0, 1.57079633, 1.57079633],
]
)
measurement = RandomizedProjectiveMeasurements(num_qubits, bias=bias, angles=angles, seed=rng)
measurement.definition().draw_bloch(
title=f"{num_qubits}-qubit Randomized Projective Measurements", colorbar=True
)
[3]:
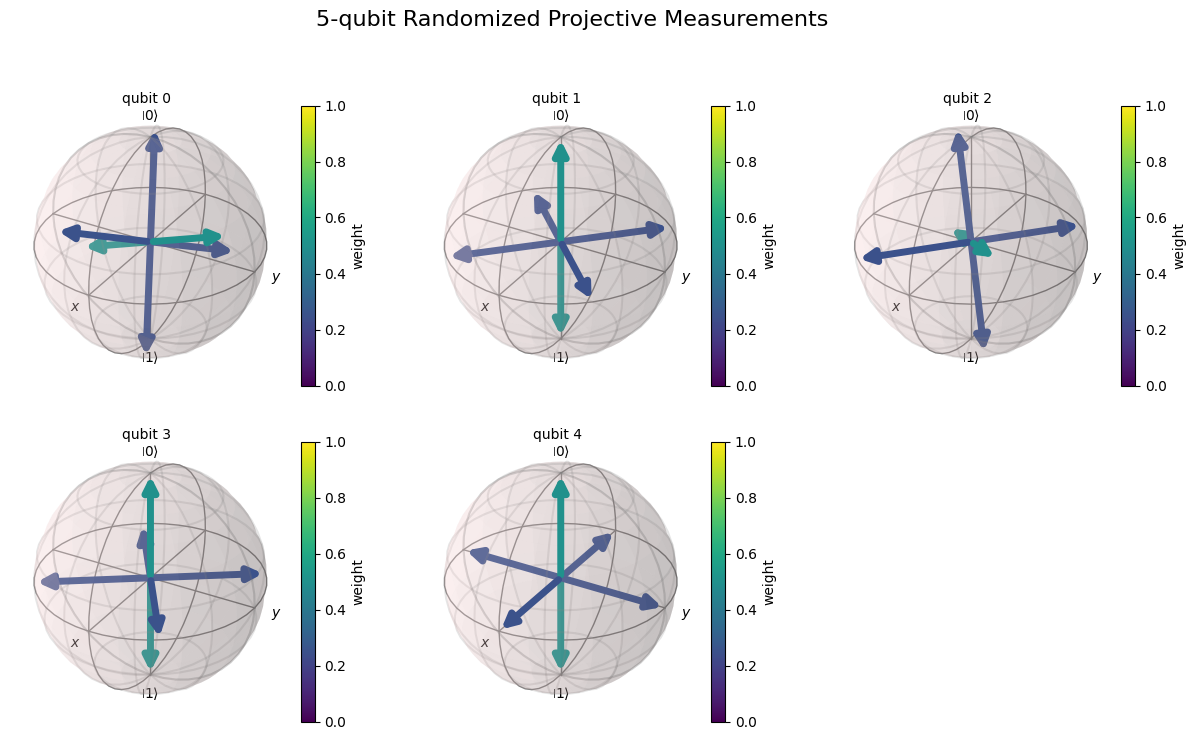
Run the job¶
Initialize Sampler
and POVMSampler
. Then run the job.
[4]:
from povm_toolbox.sampler import POVMSampler
from qiskit.primitives import StatevectorSampler
sampler = StatevectorSampler(seed=rng)
povm_sampler = POVMSampler(sampler=sampler)
job = povm_sampler.run([qc], shots=4096, povm=measurement)
pub_result = job.result()[0]
Define observable¶
[5]:
from qiskit.quantum_info import SparsePauliOp
observable = SparsePauliOp(
["XIIII", "IIYII", num_qubits * "Y", num_qubits * "Z"], coeffs=[1.3, 1.2, -1, 1.4]
)
Choosing the dual frame¶
Given a POVM
where the parametrized frame super-operator
for parameters
Note that scaling all the parameters by a fixed coefficient results in the same dual frame.
State-average dual frame (or canonical estimators)¶
The default case implemented in the POVMPostProcessor
is to use the state-average dual frame, which is defined by parameters
It is optimal with respect to the fully-mixed state
The operators of the state-average dual frame are sometimes referred to as the canonical estimators.
[6]:
from povm_toolbox.post_processor import POVMPostProcessor
from qiskit.quantum_info import Statevector
exact_expectation_value = np.real_if_close(Statevector(qc).expectation_value(observable))
print(f"Exact value: {exact_expectation_value}")
post_processor = POVMPostProcessor(pub_result)
exp_value_canonical, std_canonical = post_processor.get_expectation_value(observable)
print(f"Estimated value: {exp_value_canonical}")
print(f"\nEstimated standard deviation of the estimator: {std_canonical}")
Exact value: 0.9786732290044555
Estimated value: 0.7885807349016069
Estimated standard deviation of the estimator: 0.40445067321743994
State-marginal dual frame¶
Here we use the state-marginal dual frame, which requires the knowledge of the sampled state
where the POVM is assumed to have a product structure. We then marginalize these outcome probabilities to ensure that the dual frame will also have a product structure. That is, we set the parameters of the dual frame to
where
The caveat of this dual frame is that we do not have access to the state
[7]:
from povm_toolbox.post_processor import dual_from_marginal_probabilities
post_processor.dual = dual_from_marginal_probabilities(
povm=post_processor.povm, state=Statevector(qc)
)
exp_value_marginal, std_marginal = post_processor.get_expectation_value(observable)
print(f"Estimated value: {exp_value_marginal}")
print(f"\nEstimated standard deviation of the estimator: {std_marginal}")
Estimated value: 0.997097414528348
Estimated standard deviation of the estimator: 0.3041460424519631
Empirical frequencies dual frame¶
We now present a dual frame that uses the same idea as for the state-marginal dual frame, but without requiring the knowledge of the state
where
To add stability of the estimator (and avoid parameters set to 0 if an outcome was not sampled), we add a bias term to the frequencies as
where
We have two edge cases:
In the limit
, we recover the state-average dual frame if .In the limit
, we recover the marginal-state dual frame (independently of ).
[8]:
from povm_toolbox.post_processor import dual_from_empirical_frequencies
post_processor.dual = dual_from_empirical_frequencies(povm_post_processor=post_processor)
exp_value_freq, std_freq = post_processor.get_expectation_value(observable)
print(f"Estimated value: {exp_value_freq}")
print(f"\nEstimated standard deviation of the estimator: {std_freq}")
Estimated value: 1.0142327046855542
Estimated standard deviation of the estimator: 0.30334996039158424
Summary¶
We finally compare the estimates obtained with the different dual frames.
[9]:
print(f"Exact value: {exact_expectation_value}\n")
print("Dual frame Estimated value Estimated std Actual error")
print("------------------------------------------------------------------------")
print(
f"canonical estimators {exp_value_canonical:>18.6f} {std_canonical:>15.6f} {abs(exp_value_canonical - exact_expectation_value):>14.6f}"
)
print(
f"marginal probabilities {exp_value_marginal:>16.6f} {std_marginal:>15.6f} {abs(exp_value_marginal - exact_expectation_value):>14.6f}"
)
print(
f"empirical frequencies {exp_value_freq:>17.6f} {std_freq:>15.6f} {abs(exp_value_freq - exact_expectation_value):>14.6f}"
)
Exact value: 0.9786732290044555
Dual frame Estimated value Estimated std Actual error
------------------------------------------------------------------------
canonical estimators 0.788581 0.404451 0.190092
marginal probabilities 0.997097 0.304146 0.018424
empirical frequencies 1.014233 0.303350 0.035559