Nota
Esta página fue generada a partir de docs/tutorials/03_european_call_option_pricing.ipynb.
Fijación de Precios de Opciones de Compra Europeas#
Introducción#
Supón una opción de compra europea con precio de ejercicio
A continuación, se utiliza un algoritmo cuántico basado en la estimación de amplitud para estimar el rendimiento esperado, es decir, el precio justo antes del descuento, para la opción:
así como la correspondiente
La aproximación de la función objetivo y una introducción general a la fijación de precios de opciones y el análisis de riesgos en computadoras cuánticas se dan en los siguientes artículos:
[1]:
import matplotlib.pyplot as plt
%matplotlib inline
import numpy as np
from qiskit import QuantumCircuit
from qiskit_algorithms import IterativeAmplitudeEstimation, EstimationProblem
from qiskit.circuit.library import LinearAmplitudeFunction
from qiskit_aer.primitives import Sampler
from qiskit_finance.circuit.library import LogNormalDistribution
Modelo de Incertidumbre#
Construimos un circuito para cargar una distribución aleatoria logarítmica normal en un estado cuántico. La distribución se trunca a un intervalo dado
donde
[2]:
# number of qubits to represent the uncertainty
num_uncertainty_qubits = 3
# parameters for considered random distribution
S = 2.0 # initial spot price
vol = 0.4 # volatility of 40%
r = 0.05 # annual interest rate of 4%
T = 40 / 365 # 40 days to maturity
# resulting parameters for log-normal distribution
mu = (r - 0.5 * vol**2) * T + np.log(S)
sigma = vol * np.sqrt(T)
mean = np.exp(mu + sigma**2 / 2)
variance = (np.exp(sigma**2) - 1) * np.exp(2 * mu + sigma**2)
stddev = np.sqrt(variance)
# lowest and highest value considered for the spot price; in between, an equidistant discretization is considered.
low = np.maximum(0, mean - 3 * stddev)
high = mean + 3 * stddev
# construct A operator for QAE for the payoff function by
# composing the uncertainty model and the objective
uncertainty_model = LogNormalDistribution(
num_uncertainty_qubits, mu=mu, sigma=sigma**2, bounds=(low, high)
)
[3]:
# plot probability distribution
x = uncertainty_model.values
y = uncertainty_model.probabilities
plt.bar(x, y, width=0.2)
plt.xticks(x, size=15, rotation=90)
plt.yticks(size=15)
plt.grid()
plt.xlabel("Spot Price at Maturity $S_T$ (\$)", size=15)
plt.ylabel("Probability ($\%$)", size=15)
plt.show()
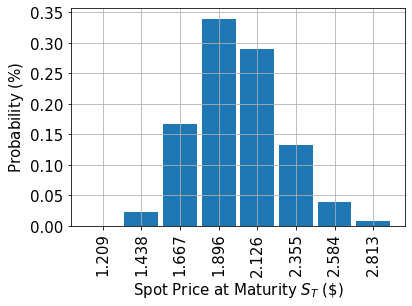
Función de Rendimiento#
La función de rendimiento es igual a cero siempre que el precio de contado al vencimiento
La parte lineal en sí es entonces aproximada de la siguiente manera. Explotamos el hecho de que
para pequeños
Podemos construir fácilmente un operador que actúe como
utilizando rotaciones Y controladas.
Finalmente, nos interesa la probabilidad de medir
Para más detalles sobre la aproximación, nos referimos a: Quantum Risk Analysis. Woerner, Egger. 2018.
[4]:
# set the strike price (should be within the low and the high value of the uncertainty)
strike_price = 1.896
# set the approximation scaling for the payoff function
c_approx = 0.25
# setup piecewise linear objective fcuntion
breakpoints = [low, strike_price]
slopes = [0, 1]
offsets = [0, 0]
f_min = 0
f_max = high - strike_price
european_call_objective = LinearAmplitudeFunction(
num_uncertainty_qubits,
slopes,
offsets,
domain=(low, high),
image=(f_min, f_max),
breakpoints=breakpoints,
rescaling_factor=c_approx,
)
# construct A operator for QAE for the payoff function by
# composing the uncertainty model and the objective
num_qubits = european_call_objective.num_qubits
european_call = QuantumCircuit(num_qubits)
european_call.append(uncertainty_model, range(num_uncertainty_qubits))
european_call.append(european_call_objective, range(num_qubits))
# draw the circuit
european_call.draw()
[4]:
┌───────┐┌────┐ q_0: ┤0 ├┤0 ├ │ ││ │ q_1: ┤1 P(X) ├┤1 ├ │ ││ │ q_2: ┤2 ├┤2 ├ └───────┘│ │ q_3: ─────────┤3 F ├ │ │ q_4: ─────────┤4 ├ │ │ q_5: ─────────┤5 ├ │ │ q_6: ─────────┤6 ├ └────┘
[5]:
# plot exact payoff function (evaluated on the grid of the uncertainty model)
x = uncertainty_model.values
y = np.maximum(0, x - strike_price)
plt.plot(x, y, "ro-")
plt.grid()
plt.title("Payoff Function", size=15)
plt.xlabel("Spot Price", size=15)
plt.ylabel("Payoff", size=15)
plt.xticks(x, size=15, rotation=90)
plt.yticks(size=15)
plt.show()
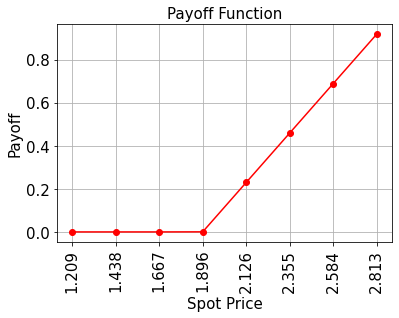
[6]:
# evaluate exact expected value (normalized to the [0, 1] interval)
exact_value = np.dot(uncertainty_model.probabilities, y)
exact_delta = sum(uncertainty_model.probabilities[x >= strike_price])
print("exact expected value:\t%.4f" % exact_value)
print("exact delta value: \t%.4f" % exact_delta)
exact expected value: 0.1623
exact delta value: 0.8098
Evaluar el Rendimiento Esperado#
[7]:
european_call.draw()
[7]:
┌───────┐┌────┐ q_0: ┤0 ├┤0 ├ │ ││ │ q_1: ┤1 P(X) ├┤1 ├ │ ││ │ q_2: ┤2 ├┤2 ├ └───────┘│ │ q_3: ─────────┤3 F ├ │ │ q_4: ─────────┤4 ├ │ │ q_5: ─────────┤5 ├ │ │ q_6: ─────────┤6 ├ └────┘
[8]:
# set target precision and confidence level
epsilon = 0.01
alpha = 0.05
problem = EstimationProblem(
state_preparation=european_call,
objective_qubits=[3],
post_processing=european_call_objective.post_processing,
)
# construct amplitude estimation
ae = IterativeAmplitudeEstimation(
epsilon_target=epsilon, alpha=alpha, sampler=Sampler(run_options={"shots": 100, "seed": 75})
)
[9]:
result = ae.estimate(problem)
[10]:
conf_int = np.array(result.confidence_interval_processed)
print("Exact value: \t%.4f" % exact_value)
print("Estimated value: \t%.4f" % (result.estimation_processed))
print("Confidence interval:\t[%.4f, %.4f]" % tuple(conf_int))
Exact value: 0.1623
Estimated value: 0.1687
Confidence interval: [0.1637, 0.1737]
En lugar de construir estos circuitos manualmente, el módulo Qiskit Finance ofrece el circuito EuropeanCallPricing
, que ya implementa esta funcionalidad como un bloque de construcción.
[11]:
from qiskit_finance.applications.estimation import EuropeanCallPricing
european_call_pricing = EuropeanCallPricing(
num_state_qubits=num_uncertainty_qubits,
strike_price=strike_price,
rescaling_factor=c_approx,
bounds=(low, high),
uncertainty_model=uncertainty_model,
)
[12]:
# set target precision and confidence level
epsilon = 0.01
alpha = 0.05
problem = european_call_pricing.to_estimation_problem()
# construct amplitude estimation
ae = IterativeAmplitudeEstimation(
epsilon_target=epsilon, alpha=alpha, sampler=Sampler(run_options={"shots": 100, "seed": 75})
)
result = ae.estimate(problem)
conf_int = np.array(result.confidence_interval_processed)
print("Exact value: \t%.4f" % exact_value)
print("Estimated value: \t%.4f" % (european_call_pricing.interpret(result)))
print("Confidence interval:\t[%.4f, %.4f]" % tuple(conf_int))
Exact value: 0.1623
Estimated value: 0.1687
Confidence interval: [0.1637, 0.1737]
Evaluar Delta#
La Delta es un poco más simple de evaluar que el rendimiento esperado. De manera similar al rendimiento esperado, usamos un circuito comparador y un qubit ancilla para identificar los casos donde
[13]:
from qiskit_finance.applications.estimation import EuropeanCallDelta
european_call_delta = EuropeanCallDelta(
num_state_qubits=num_uncertainty_qubits,
strike_price=strike_price,
bounds=(low, high),
uncertainty_model=uncertainty_model,
)
[14]:
european_call_delta._objective.decompose().draw()
[14]:
┌──────┐ state_0: ┤0 ├ │ │ state_1: ┤1 ├ │ │ state_2: ┤2 ├ │ cmp │ state_3: ┤3 ├ │ │ work_0: ┤4 ├ │ │ work_1: ┤5 ├ └──────┘
[15]:
european_call_delta_circ = QuantumCircuit(european_call_delta._objective.num_qubits)
european_call_delta_circ.append(uncertainty_model, range(num_uncertainty_qubits))
european_call_delta_circ.append(
european_call_delta._objective, range(european_call_delta._objective.num_qubits)
)
european_call_delta_circ.draw()
[15]:
┌───────┐┌──────┐ q_0: ┤0 ├┤0 ├ │ ││ │ q_1: ┤1 P(X) ├┤1 ├ │ ││ │ q_2: ┤2 ├┤2 ├ └───────┘│ ECD │ q_3: ─────────┤3 ├ │ │ q_4: ─────────┤4 ├ │ │ q_5: ─────────┤5 ├ └──────┘
[16]:
# set target precision and confidence level
epsilon = 0.01
alpha = 0.05
problem = european_call_delta.to_estimation_problem()
# construct amplitude estimation
ae_delta = IterativeAmplitudeEstimation(
epsilon_target=epsilon, alpha=alpha, sampler=Sampler(run_options={"shots": 100, "seed": 75})
)
[17]:
result_delta = ae_delta.estimate(problem)
[18]:
conf_int = np.array(result_delta.confidence_interval_processed)
print("Exact delta: \t%.4f" % exact_delta)
print("Estimated value: \t%.4f" % european_call_delta.interpret(result_delta))
print("Confidence interval: \t[%.4f, %.4f]" % tuple(conf_int))
Exact delta: 0.8098
Estimated value: 0.8091
Confidence interval: [0.8034, 0.8148]
[19]:
import qiskit.tools.jupyter
%qiskit_version_table
%qiskit_copyright
Version Information
Software | Version |
---|---|
qiskit | None |
qiskit-terra | 0.45.0.dev0+c626be7 |
qiskit_finance | 0.4.0 |
qiskit_aer | 0.12.0 |
qiskit_algorithms | 0.2.0 |
qiskit_ibm_provider | 0.6.1 |
qiskit_optimization | 0.6.0 |
System information | |
Python version | 3.9.7 |
Python compiler | GCC 7.5.0 |
Python build | default, Sep 16 2021 13:09:58 |
OS | Linux |
CPUs | 2 |
Memory (Gb) | 5.778430938720703 |
Fri Aug 18 16:00:58 2023 EDT |
This code is a part of Qiskit
© Copyright IBM 2017, 2023.
This code is licensed under the Apache License, Version 2.0. You may
obtain a copy of this license in the LICENSE.txt file in the root directory
of this source tree or at http://www.apache.org/licenses/LICENSE-2.0.
Any modifications or derivative works of this code must retain this
copyright notice, and modified files need to carry a notice indicating
that they have been altered from the originals.
[ ]: