নোট
এই পৃষ্ঠাটি docs/tutorials/03_european_call_option_pricing.ipynb থেকে বানানো হয়েছে।
ইউরোপীয় কল বিকল্পগুলির মূল্য নির্ধারণ#
ভূমিকা#
মনে করা যাক স্ট্রাইক প্রাইস সহ একটি ইউরোপীয় পুট বিকল্প \(K\) এবং একটি অন্তর্নিহিত সম্পদ যার পূর্ণ পরিণতিতে স্পট মূল্য \(S_T\), যেটি একটি প্রদত্ত এলোমেলো বিতরণ অনুসরণ করে। এই সম্পর্কিত পে অফ ফাংশনটি সংজ্ঞায়িত করা হয়:
নিম্নে একটি মানের (অ্যামপ্লিটিউড) উপর নির্ভরশীল কোয়ান্টাম অ্যালগোরিদম ব্যবহার করে প্রত্যাশিত পেঅফ, যা হলো ছাড় দেয়ার আগের আদর্শমূল্য, অনুমান করা হয়েছে।
একই সাথে সম্পর্কযুক্ত \(\Delta\) অর্থাৎ স্পট দামের সাপেক্ষে বিকল্প দামের অন্তরকলন এভাবে সংজ্ঞায়িত:
অবজেক্টিভ ফাংশনের হিসাব এবং কোয়ান্টাম কম্পিউটারগুলিতে মূল্য নির্ধারণ এবং ঝুঁকি বিশ্লেষণের একটি সাধারণ ভূমিকা নিম্নলিখিত গবেষণাপত্রগুলোতে দেওয়া হয়েছে:
[1]:
import matplotlib.pyplot as plt
%matplotlib inline
import numpy as np
from qiskit import QuantumCircuit
from qiskit_algorithms import IterativeAmplitudeEstimation, EstimationProblem
from qiskit.circuit.library import LinearAmplitudeFunction
from qiskit_aer.primitives import Sampler
from qiskit_finance.circuit.library import LogNormalDistribution
অনিশ্চয়তা মডেল#
We construct a circuit to load a log-normal random distribution into a quantum state. The distribution is truncated to a given interval \([\text{low}, \text{high}]\) and discretized using \(2^n\) grid points, where \(n\) denotes the number of qubits used. The unitary operator corresponding to the circuit implements the following:
যেখানে math:p_i কাটা এবং বিযুক্ত বিতরণগুলির সম্ভাবনা বোঝায় এবং \(i\) এর সাদৃশ্যপূর্ণ ম্যাপ ব্যবহার করে ডান ব্যবধানে ম্যাপ করা হয়েছে:
[2]:
# number of qubits to represent the uncertainty
num_uncertainty_qubits = 3
# parameters for considered random distribution
S = 2.0 # initial spot price
vol = 0.4 # volatility of 40%
r = 0.05 # annual interest rate of 4%
T = 40 / 365 # 40 days to maturity
# resulting parameters for log-normal distribution
mu = (r - 0.5 * vol**2) * T + np.log(S)
sigma = vol * np.sqrt(T)
mean = np.exp(mu + sigma**2 / 2)
variance = (np.exp(sigma**2) - 1) * np.exp(2 * mu + sigma**2)
stddev = np.sqrt(variance)
# lowest and highest value considered for the spot price; in between, an equidistant discretization is considered.
low = np.maximum(0, mean - 3 * stddev)
high = mean + 3 * stddev
# construct A operator for QAE for the payoff function by
# composing the uncertainty model and the objective
uncertainty_model = LogNormalDistribution(
num_uncertainty_qubits, mu=mu, sigma=sigma**2, bounds=(low, high)
)
[3]:
# plot probability distribution
x = uncertainty_model.values
y = uncertainty_model.probabilities
plt.bar(x, y, width=0.2)
plt.xticks(x, size=15, rotation=90)
plt.yticks(size=15)
plt.grid()
plt.xlabel("Spot Price at Maturity $S_T$ (\$)", size=15)
plt.ylabel("Probability ($\%$)", size=15)
plt.show()
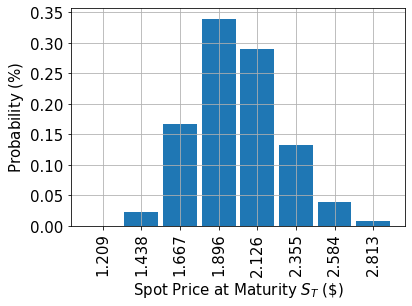
পেঅফ ফাংশন#
যতক্ষণ পরিপক্ক অবস্থায় স্ট্রাইক মূল্য \(S_T\), \(K\) থেকে কম থাকছে ততক্ষণ পর্যন্ত পেঅফ ফাংশন শূন্য থাকবে তারপর মান রৈখিকভাবে বৃদ্ধি পাবে। এই প্রয়োগপদ্ধতিতে একটি comparator ব্যবহার করা হয়, এটি \(\big|0\rangle\) থেকে \(\big|1\rangle\) পর্যন্ত যদি \(S_T \geq K\) হয় তাহলে একটি অ্যানসিলা কিউবিট ফ্লিপ করে এবং অ্যানসিলাটি পে অফ ফাঙ্কশন এর লিনিয়ার অংশ নিয়ন্ত্রণ করতে ব্যবহৃত হয়।
রৈখিক অংশ (linear part) টি অনুমান করতে এই পদ্ধতি ব্যবহার করা হয়। ছোট \(|y|\) এর মানের জন্যে \(\sin^2(y + \pi/4) \approx y + 1/2\) হয়। ফলে যেকোনো প্রদত্ত আনুমানিক আরোহী গুণক (স্কেলিং factor) \(c_\text{approx} \in [0, 1]\) এবং \(x \in [0, 1]\) এর জন্যে আমরা ধরে নেই,
ছোট :math:`c_text{approx}`এর জন্যে।
আমরা খুব সহজেই একটি চালক বানাতে পারি যেটা
নিয়ন্ত্রিত Y-রোটেশন ব্যবহার করে
অবশেষে, আমরা শেষ কিউবিটে \(\big|1\rangle\) সম্ভাবনা পরিমাপে আগ্রহী, যা \(\sin^2(a*x+b)\) এর সাথে মিলে যাবার কথা। এই অনুমান গুলোর সাহায্যে আমরা এক্সপেক্টেশন মানগুলির নিকটবর্তী মাত্রাগুলো পেয়ে যাব। যত ছোট \(c_{approx}\) এর মান হবে, আমাদের প্রাপ্ত অনুমান তত ভালো হবে। কিন্তু এখানে এটাও মাথায় রাখা দরকার যে যেহেতু অনুমানটি \(c_{approx}\) এর ওপরে নির্ভর তাই মূল্যায়নের কিউবিটগুলোর সংখ্যা \(m\) সেই অনুসারে সামঞ্জস্য করা দরকার।
অনুমান সম্পর্কিত বিস্তারিত জানার জন্য এই গবেষণাপত্রটি দেখো: Quantum Risk Analysis. Woerner, Egger. 2018.
[4]:
# set the strike price (should be within the low and the high value of the uncertainty)
strike_price = 1.896
# set the approximation scaling for the payoff function
c_approx = 0.25
# setup piecewise linear objective fcuntion
breakpoints = [low, strike_price]
slopes = [0, 1]
offsets = [0, 0]
f_min = 0
f_max = high - strike_price
european_call_objective = LinearAmplitudeFunction(
num_uncertainty_qubits,
slopes,
offsets,
domain=(low, high),
image=(f_min, f_max),
breakpoints=breakpoints,
rescaling_factor=c_approx,
)
# construct A operator for QAE for the payoff function by
# composing the uncertainty model and the objective
num_qubits = european_call_objective.num_qubits
european_call = QuantumCircuit(num_qubits)
european_call.append(uncertainty_model, range(num_uncertainty_qubits))
european_call.append(european_call_objective, range(num_qubits))
# draw the circuit
european_call.draw()
[4]:
┌───────┐┌────┐ q_0: ┤0 ├┤0 ├ │ ││ │ q_1: ┤1 P(X) ├┤1 ├ │ ││ │ q_2: ┤2 ├┤2 ├ └───────┘│ │ q_3: ─────────┤3 F ├ │ │ q_4: ─────────┤4 ├ │ │ q_5: ─────────┤5 ├ │ │ q_6: ─────────┤6 ├ └────┘
[5]:
# plot exact payoff function (evaluated on the grid of the uncertainty model)
x = uncertainty_model.values
y = np.maximum(0, x - strike_price)
plt.plot(x, y, "ro-")
plt.grid()
plt.title("Payoff Function", size=15)
plt.xlabel("Spot Price", size=15)
plt.ylabel("Payoff", size=15)
plt.xticks(x, size=15, rotation=90)
plt.yticks(size=15)
plt.show()
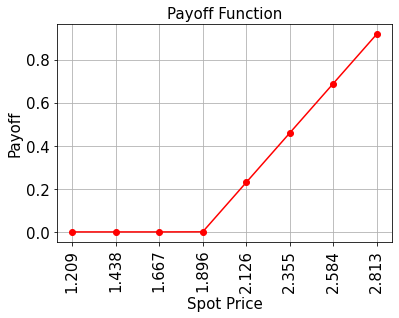
[6]:
# evaluate exact expected value (normalized to the [0, 1] interval)
exact_value = np.dot(uncertainty_model.probabilities, y)
exact_delta = sum(uncertainty_model.probabilities[x >= strike_price])
print("exact expected value:\t%.4f" % exact_value)
print("exact delta value: \t%.4f" % exact_delta)
exact expected value: 0.1623
exact delta value: 0.8098
প্রত্যাশিত পেঅফ নির্ধারণ করা#
[7]:
european_call.draw()
[7]:
┌───────┐┌────┐ q_0: ┤0 ├┤0 ├ │ ││ │ q_1: ┤1 P(X) ├┤1 ├ │ ││ │ q_2: ┤2 ├┤2 ├ └───────┘│ │ q_3: ─────────┤3 F ├ │ │ q_4: ─────────┤4 ├ │ │ q_5: ─────────┤5 ├ │ │ q_6: ─────────┤6 ├ └────┘
[8]:
# set target precision and confidence level
epsilon = 0.01
alpha = 0.05
problem = EstimationProblem(
state_preparation=european_call,
objective_qubits=[3],
post_processing=european_call_objective.post_processing,
)
# construct amplitude estimation
ae = IterativeAmplitudeEstimation(
epsilon_target=epsilon, alpha=alpha, sampler=Sampler(run_options={"shots": 100, "seed": 75})
)
[9]:
result = ae.estimate(problem)
[10]:
conf_int = np.array(result.confidence_interval_processed)
print("Exact value: \t%.4f" % exact_value)
print("Estimated value: \t%.4f" % (result.estimation_processed))
print("Confidence interval:\t[%.4f, %.4f]" % tuple(conf_int))
Exact value: 0.1623
Estimated value: 0.1687
Confidence interval: [0.1637, 0.1737]
Instead of constructing these circuits manually, the Qiskit Finance module offers the EuropeanCallPricing
circuit, which already implements this functionality as a building block.
[11]:
from qiskit_finance.applications.estimation import EuropeanCallPricing
european_call_pricing = EuropeanCallPricing(
num_state_qubits=num_uncertainty_qubits,
strike_price=strike_price,
rescaling_factor=c_approx,
bounds=(low, high),
uncertainty_model=uncertainty_model,
)
[12]:
# set target precision and confidence level
epsilon = 0.01
alpha = 0.05
problem = european_call_pricing.to_estimation_problem()
# construct amplitude estimation
ae = IterativeAmplitudeEstimation(
epsilon_target=epsilon, alpha=alpha, sampler=Sampler(run_options={"shots": 100, "seed": 75})
)
result = ae.estimate(problem)
conf_int = np.array(result.confidence_interval_processed)
print("Exact value: \t%.4f" % exact_value)
print("Estimated value: \t%.4f" % (european_call_pricing.interpret(result)))
print("Confidence interval:\t[%.4f, %.4f]" % tuple(conf_int))
Exact value: 0.1623
Estimated value: 0.1687
Confidence interval: [0.1637, 0.1737]
ডেল্টা মূল্যায়ন করা#
প্রত্যাশিত পেঅফের চেয়ে ডেল্টা মূল্যায়ন করা কিছুটা সহজ। প্রত্যাশিত পেঅফ এর মতোই আমরা \(S_T \leq K\) কেসগুলি সনাক্ত করতে একটি তুলনামূলক বর্তনী (সার্কিট) এবং একটি অ্যানসিলা কিউবিট ব্যবহার করি। তবে, যেহেতু আমরা কেবলমাত্র এই অবস্থা সত্য হওয়ার সম্ভাবনা সম্পর্কে আগ্রহী, তাই আমরা আরও কিছু অনুমান ছাড়াই সরাসরি বিস্তার অনুমানে এই অ্যানসিলা কিউবিট টি অবজেক্টিভ কিউবিট হিসাবে ব্যবহার করতে পারি।
[13]:
from qiskit_finance.applications.estimation import EuropeanCallDelta
european_call_delta = EuropeanCallDelta(
num_state_qubits=num_uncertainty_qubits,
strike_price=strike_price,
bounds=(low, high),
uncertainty_model=uncertainty_model,
)
[14]:
european_call_delta._objective.decompose().draw()
[14]:
┌──────┐ state_0: ┤0 ├ │ │ state_1: ┤1 ├ │ │ state_2: ┤2 ├ │ cmp │ state_3: ┤3 ├ │ │ work_0: ┤4 ├ │ │ work_1: ┤5 ├ └──────┘
[15]:
european_call_delta_circ = QuantumCircuit(european_call_delta._objective.num_qubits)
european_call_delta_circ.append(uncertainty_model, range(num_uncertainty_qubits))
european_call_delta_circ.append(
european_call_delta._objective, range(european_call_delta._objective.num_qubits)
)
european_call_delta_circ.draw()
[15]:
┌───────┐┌──────┐ q_0: ┤0 ├┤0 ├ │ ││ │ q_1: ┤1 P(X) ├┤1 ├ │ ││ │ q_2: ┤2 ├┤2 ├ └───────┘│ ECD │ q_3: ─────────┤3 ├ │ │ q_4: ─────────┤4 ├ │ │ q_5: ─────────┤5 ├ └──────┘
[16]:
# set target precision and confidence level
epsilon = 0.01
alpha = 0.05
problem = european_call_delta.to_estimation_problem()
# construct amplitude estimation
ae_delta = IterativeAmplitudeEstimation(
epsilon_target=epsilon, alpha=alpha, sampler=Sampler(run_options={"shots": 100, "seed": 75})
)
[17]:
result_delta = ae_delta.estimate(problem)
[18]:
conf_int = np.array(result_delta.confidence_interval_processed)
print("Exact delta: \t%.4f" % exact_delta)
print("Estimated value: \t%.4f" % european_call_delta.interpret(result_delta))
print("Confidence interval: \t[%.4f, %.4f]" % tuple(conf_int))
Exact delta: 0.8098
Estimated value: 0.8091
Confidence interval: [0.8034, 0.8148]
[19]:
import qiskit.tools.jupyter
%qiskit_version_table
%qiskit_copyright
Version Information
Software | Version |
---|---|
qiskit | None |
qiskit-terra | 0.45.0.dev0+c626be7 |
qiskit_finance | 0.4.0 |
qiskit_aer | 0.12.0 |
qiskit_algorithms | 0.2.0 |
qiskit_ibm_provider | 0.6.1 |
qiskit_optimization | 0.6.0 |
System information | |
Python version | 3.9.7 |
Python compiler | GCC 7.5.0 |
Python build | default, Sep 16 2021 13:09:58 |
OS | Linux |
CPUs | 2 |
Memory (Gb) | 5.778430938720703 |
Fri Aug 18 16:00:58 2023 EDT |
This code is a part of Qiskit
© Copyright IBM 2017, 2023.
This code is licensed under the Apache License, Version 2.0. You may
obtain a copy of this license in the LICENSE.txt file in the root directory
of this source tree or at http://www.apache.org/licenses/LICENSE-2.0.
Any modifications or derivative works of this code must retain this
copyright notice, and modified files need to carry a notice indicating
that they have been altered from the originals.
[ ]: