নোট
এই পেজটি docs/tutorials/09_credit_risk_analysis.ipynb থেকে তৈরি হয়েছে।
ক্রেডিট ঝুঁকি বিশ্লেষণ#
ভূমিকা#
এই টিউটোরিয়াল দেখায় কিভাবে ক্রেডিট ঝুঁকি বিশ্লেষণের জন্য কোয়ান্টাম অ্যালগরিদম ব্যবহার করা যায়। আরো স্পষ্টভাবে, কিভাবে কোয়ান্টাম প্রশস্ততা অনুমান (QAE) প্রথাগত (ক্লাসিক্যাল) মন্টে কার্লো সিমুলেশন উপর একটি দ্বিঘাত গতি বৃদ্ধির সঙ্গে ঝুঁকি পরিমাপ অনুমান করতে ব্যবহার করা যেতে পারে। টিউটোরিয়ালটি নিম্নলিখিত গবেষণার উপর ভিত্তি করে:
Quantum Risk Analysis. Stefan Woerner, Daniel J. Egger. [Woerner2019]
Credit Risk Analysis using Quantum Computers. Egger et al. (2019) [Egger2019]
QAE- এর একটি সাধারণ ভূমিকা নিম্নলিখিত গবেষণায় পাওয়া যাবে:
টিউটোরিয়ালের গঠন নিম্নরূপ:
Problem Definition
Uncertainty Model
Expected Loss
Cumulative Distribution Function
Value at Risk
Conditional Value at Risk
[1]:
import numpy as np
import matplotlib.pyplot as plt
from qiskit import QuantumRegister, QuantumCircuit
from qiskit.circuit.library import IntegerComparator
from qiskit_algorithms import IterativeAmplitudeEstimation, EstimationProblem
from qiskit_aer.primitives import Sampler
সমস্যার সংজ্ঞায়ণ#
এই টিউটোরিয়ালে আমরা
যেখানে
আমরা মোট লোকসানের ঝুঁকিপূর্ণতা বিশ্লেষণ করতে আগ্রহী
যেখানে
যার confidence level
বিবেচিত মডেল সম্পর্কে আরো বিস্তারিত জানার জন্য, দেখুন, যেমন, Regulatory Capital Modeling for Credit Risk. Marek Rutkowski, Silvio Tarca
The problem is defined by the following parameters:
number of qubits used to represent
, denoted bytruncation value for
, denoted by , i.e., Z is assumed to take equidistant values inthe base default probabilities for each asset
,sensitivities of the default probabilities with respect to
, denoted byloss given default for asset
, denoted byconfidence level for VaR / CVaR
.
[2]:
# set problem parameters
n_z = 2
z_max = 2
z_values = np.linspace(-z_max, z_max, 2**n_z)
p_zeros = [0.15, 0.25]
rhos = [0.1, 0.05]
lgd = [1, 2]
K = len(p_zeros)
alpha = 0.05
অনিশ্চয়তা মডেল#
আমরা এখন একটি সার্কিট তৈরি করি যা অনিশ্চয়তা মডেল লোড করে।
যেখানে আমরা
[3]:
from qiskit_finance.circuit.library import GaussianConditionalIndependenceModel as GCI
u = GCI(n_z, z_max, p_zeros, rhos)
[4]:
u.draw()
[4]:
┌───────┐ q_0: ┤0 ├ │ │ q_1: ┤1 ├ │ P(X) │ q_2: ┤2 ├ │ │ q_3: ┤3 ├ └───────┘
We now use the simulator to validate the circuit that constructs
expected loss
PDF and CDF of
value at risk
and corresponding probabilityconditional value at risk
[5]:
u_measure = u.measure_all(inplace=False)
sampler = Sampler()
job = sampler.run(u_measure)
binary_probabilities = job.result().quasi_dists[0].binary_probabilities()
[6]:
# analyze uncertainty circuit and determine exact solutions
p_z = np.zeros(2**n_z)
p_default = np.zeros(K)
values = []
probabilities = []
num_qubits = u.num_qubits
for i, prob in binary_probabilities.items():
# extract value of Z and corresponding probability
i_normal = int(i[-n_z:], 2)
p_z[i_normal] += prob
# determine overall default probability for k
loss = 0
for k in range(K):
if i[K - k - 1] == "1":
p_default[k] += prob
loss += lgd[k]
values += [loss]
probabilities += [prob]
values = np.array(values)
probabilities = np.array(probabilities)
expected_loss = np.dot(values, probabilities)
losses = np.sort(np.unique(values))
pdf = np.zeros(len(losses))
for i, v in enumerate(losses):
pdf[i] += sum(probabilities[values == v])
cdf = np.cumsum(pdf)
i_var = np.argmax(cdf >= 1 - alpha)
exact_var = losses[i_var]
exact_cvar = np.dot(pdf[(i_var + 1) :], losses[(i_var + 1) :]) / sum(pdf[(i_var + 1) :])
[7]:
print("Expected Loss E[L]: %.4f" % expected_loss)
print("Value at Risk VaR[L]: %.4f" % exact_var)
print("P[L <= VaR[L]]: %.4f" % cdf[exact_var])
print("Conditional Value at Risk CVaR[L]: %.4f" % exact_cvar)
Expected Loss E[L]: 0.6641
Value at Risk VaR[L]: 2.0000
P[L <= VaR[L]]: 0.9521
Conditional Value at Risk CVaR[L]: 3.0000
[8]:
# plot loss PDF, expected loss, var, and cvar
plt.bar(losses, pdf)
plt.axvline(expected_loss, color="green", linestyle="--", label="E[L]")
plt.axvline(exact_var, color="orange", linestyle="--", label="VaR(L)")
plt.axvline(exact_cvar, color="red", linestyle="--", label="CVaR(L)")
plt.legend(fontsize=15)
plt.xlabel("Loss L ($)", size=15)
plt.ylabel("probability (%)", size=15)
plt.title("Loss Distribution", size=20)
plt.xticks(size=15)
plt.yticks(size=15)
plt.show()
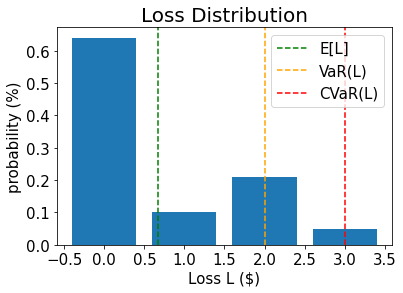
[9]:
# plot results for Z
plt.plot(z_values, p_z, "o-", linewidth=3, markersize=8)
plt.grid()
plt.xlabel("Z value", size=15)
plt.ylabel("probability (%)", size=15)
plt.title("Z Distribution", size=20)
plt.xticks(size=15)
plt.yticks(size=15)
plt.show()
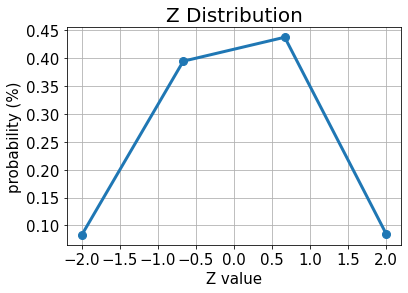
[10]:
# plot results for default probabilities
plt.bar(range(K), p_default)
plt.xlabel("Asset", size=15)
plt.ylabel("probability (%)", size=15)
plt.title("Individual Default Probabilities", size=20)
plt.xticks(range(K), size=15)
plt.yticks(size=15)
plt.grid()
plt.show()
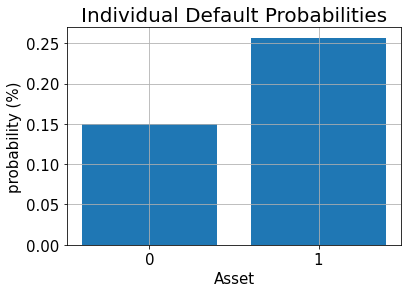
গড় লোকসান#
গড় লোকসান অনুমান করতে, আমরা প্রথমে ওজনযুক্ত সমষ্টি অপারেটরটি প্রয়োগ করে পৃথক লোকসান সমষ্টি করি।
ফলাফল বর্ণনা করার জন্য প্রয়োজনীয় কিউবিটগুলো হল
একবার আমাদের একটি কোয়ান্টাম রেজিস্টারে মোট ক্ষতি বন্টন হয়ে গেলে, আমরা [Woerner2019] এ বর্ণিত কৌশলগুলি ব্যবহার করে মোট ক্ষতির চিত্রায়ন তৈরি করতে পারি
যা গড় লোকসান মূল্যায়ন করতে বিস্তার অনুমান চালাতে দেয়।
[11]:
# add Z qubits with weight/loss 0
from qiskit.circuit.library import WeightedAdder
agg = WeightedAdder(n_z + K, [0] * n_z + lgd)
[12]:
from qiskit.circuit.library import LinearAmplitudeFunction
# define linear objective function
breakpoints = [0]
slopes = [1]
offsets = [0]
f_min = 0
f_max = sum(lgd)
c_approx = 0.25
objective = LinearAmplitudeFunction(
agg.num_sum_qubits,
slope=slopes,
offset=offsets,
# max value that can be reached by the qubit register (will not always be reached)
domain=(0, 2**agg.num_sum_qubits - 1),
image=(f_min, f_max),
rescaling_factor=c_approx,
breakpoints=breakpoints,
)
মান বা অবস্থা প্রস্তুতিকরন বর্তনী তৈরি কর:
[13]:
# define the registers for convenience and readability
qr_state = QuantumRegister(u.num_qubits, "state")
qr_sum = QuantumRegister(agg.num_sum_qubits, "sum")
qr_carry = QuantumRegister(agg.num_carry_qubits, "carry")
qr_obj = QuantumRegister(1, "objective")
# define the circuit
state_preparation = QuantumCircuit(qr_state, qr_obj, qr_sum, qr_carry, name="A")
# load the random variable
state_preparation.append(u.to_gate(), qr_state)
# aggregate
state_preparation.append(agg.to_gate(), qr_state[:] + qr_sum[:] + qr_carry[:])
# linear objective function
state_preparation.append(objective.to_gate(), qr_sum[:] + qr_obj[:])
# uncompute aggregation
state_preparation.append(agg.to_gate().inverse(), qr_state[:] + qr_sum[:] + qr_carry[:])
# draw the circuit
state_preparation.draw()
[13]:
┌───────┐┌────────┐ ┌───────────┐ state_0: ┤0 ├┤0 ├──────┤0 ├ │ ││ │ │ │ state_1: ┤1 ├┤1 ├──────┤1 ├ │ P(X) ││ │ │ │ state_2: ┤2 ├┤2 ├──────┤2 ├ │ ││ │ │ │ state_3: ┤3 ├┤3 ├──────┤3 ├ └───────┘│ adder │┌────┐│ adder_dg │ objective: ─────────┤ ├┤2 ├┤ ├ │ ││ ││ │ sum_0: ─────────┤4 ├┤0 F ├┤4 ├ │ ││ ││ │ sum_1: ─────────┤5 ├┤1 ├┤5 ├ │ │└────┘│ │ carry: ─────────┤6 ├──────┤6 ├ └────────┘ └───────────┘
আমরা গড় ক্ষতির অনুমান করার জন্য QAE ব্যবহার করার আগে, আমরা কোয়ান্টাম সার্কিটকে অব্জেক্টিভ ফাংশনকে প্রতিনিধিত্ব করে কেবলমাত্র এটিকে সরাসরি অনুকরণ করে এবং
[14]:
state_preparation_measure = state_preparation.measure_all(inplace=False)
sampler = Sampler()
job = sampler.run(state_preparation_measure)
binary_probabilities = job.result().quasi_dists[0].binary_probabilities()
[15]:
# evaluate the result
value = 0
for i, prob in binary_probabilities.items():
if prob > 1e-6 and i[-(len(qr_state) + 1) :][0] == "1":
value += prob
print("Exact Expected Loss: %.4f" % expected_loss)
print("Exact Operator Value: %.4f" % value)
print("Mapped Operator value: %.4f" % objective.post_processing(value))
Exact Expected Loss: 0.6641
Exact Operator Value: 0.3789
Mapped Operator value: 0.5749
পরবর্তীতে আমরা গড় ক্ষতি অনুমান করতে QAE চালাচ্ছি ক্লাসিকাল Monte Carlo সিমুলেশনের উপর দ্বিঘাত স্পীড-আপ দিয়ে।
[16]:
# set target precision and confidence level
epsilon = 0.01
alpha = 0.05
problem = EstimationProblem(
state_preparation=state_preparation,
objective_qubits=[len(qr_state)],
post_processing=objective.post_processing,
)
# construct amplitude estimation
ae = IterativeAmplitudeEstimation(
epsilon_target=epsilon, alpha=alpha, sampler=Sampler(run_options={"shots": 100, "seed": 75})
)
result = ae.estimate(problem)
# print results
conf_int = np.array(result.confidence_interval_processed)
print("Exact value: \t%.4f" % expected_loss)
print("Estimated value:\t%.4f" % result.estimation_processed)
print("Confidence interval: \t[%.4f, %.4f]" % tuple(conf_int))
Exact value: 0.6641
Estimated value: 0.6863
Confidence interval: [0.6175, 0.7552]
ক্রমবর্ধমান বন্টন ফাংশন#
গড় ক্ষতির পরিবর্তে (যা ক্লাসিকাল কৌশলগুলি ব্যবহার করেও দক্ষতার সাথে অনুমান করা যেতে পারে) আমরা এখন ক্ষতির ক্রম বিতরণ ফাংশন (cumulative distribution function বা সংক্ষেপে CDF) নিরূপন করি। প্রথাগতভাবে, হয় এটি সমস্ত খেলাপি সম্পদের সম্ভাব্য সমাবেশের মূল্যায়ন করা হয়, অথবা অনেকগুলো প্রথাগত (ক্লাসিক্যাল) স্যাম্পেলের মূল্যায়ন মন্টি কার্লো সিমুলেশনের মাধ্যমে করা হয়। ভবিষ্যতে QAE ভিত্তিক অ্যালগরিদমের (ধারাক্রমের) এইসকল বিশ্লেষণের গতি উল্লেখযোগ্যভাবে বৃদ্ধি পাওয়ার সম্ভাবনা রয়েছে।
CDF, অর্থাৎ সম্ভাব্যতা
ফলে কোয়ান্টাম অবস্থাকে লেখা যায়
যেখানে আমরা অনিশ্চয়তার মডেলটির বিশদ উপস্থাপনের পরিবর্তে সরাসরি সমষ্টি করা লোকসানের মানগুলি এবং সম্পর্কিত সম্ভাব্যতাগুলি ধরে নিই।
The CDF(
[17]:
# set x value to estimate the CDF
x_eval = 2
comparator = IntegerComparator(agg.num_sum_qubits, x_eval + 1, geq=False)
comparator.draw()
[17]:
┌──────┐ state_0: ┤0 ├ │ │ state_1: ┤1 ├ │ cmp │ compare: ┤2 ├ │ │ a15: ┤3 ├ └──────┘
[18]:
def get_cdf_circuit(x_eval):
# define the registers for convenience and readability
qr_state = QuantumRegister(u.num_qubits, "state")
qr_sum = QuantumRegister(agg.num_sum_qubits, "sum")
qr_carry = QuantumRegister(agg.num_carry_qubits, "carry")
qr_obj = QuantumRegister(1, "objective")
qr_compare = QuantumRegister(1, "compare")
# define the circuit
state_preparation = QuantumCircuit(qr_state, qr_obj, qr_sum, qr_carry, name="A")
# load the random variable
state_preparation.append(u, qr_state)
# aggregate
state_preparation.append(agg, qr_state[:] + qr_sum[:] + qr_carry[:])
# comparator objective function
comparator = IntegerComparator(agg.num_sum_qubits, x_eval + 1, geq=False)
state_preparation.append(comparator, qr_sum[:] + qr_obj[:] + qr_carry[:])
# uncompute aggregation
state_preparation.append(agg.inverse(), qr_state[:] + qr_sum[:] + qr_carry[:])
return state_preparation
state_preparation = get_cdf_circuit(x_eval)
আবার আমরা প্রথমে কোয়ান্টাম সিমুলেশন ব্যবহার করব কোয়ান্টাম বর্তনী যাচাইয়ের জন্য।
[19]:
state_preparation.draw()
[19]:
┌───────┐┌────────┐ ┌───────────┐ state_0: ┤0 ├┤0 ├────────┤0 ├ │ ││ │ │ │ state_1: ┤1 ├┤1 ├────────┤1 ├ │ P(X) ││ │ │ │ state_2: ┤2 ├┤2 ├────────┤2 ├ │ ││ │ │ │ state_3: ┤3 ├┤3 ├────────┤3 ├ └───────┘│ adder │┌──────┐│ adder_dg │ objective: ─────────┤ ├┤2 ├┤ ├ │ ││ ││ │ sum_0: ─────────┤4 ├┤0 ├┤4 ├ │ ││ cmp ││ │ sum_1: ─────────┤5 ├┤1 ├┤5 ├ │ ││ ││ │ carry: ─────────┤6 ├┤3 ├┤6 ├ └────────┘└──────┘└───────────┘
[20]:
state_preparation_measure = state_preparation.measure_all(inplace=False)
sampler = Sampler()
job = sampler.run(state_preparation_measure)
binary_probabilities = job.result().quasi_dists[0].binary_probabilities()
[21]:
# evaluate the result
var_prob = 0
for i, prob in binary_probabilities.items():
if prob > 1e-6 and i[-(len(qr_state) + 1) :][0] == "1":
var_prob += prob
print("Operator CDF(%s)" % x_eval + " = %.4f" % var_prob)
print("Exact CDF(%s)" % x_eval + " = %.4f" % cdf[x_eval])
Operator CDF(2) = 0.9551
Exact CDF(2) = 0.9521
এরপরে আমরা CDF অনুমানের জন্য QAE চালাই
[22]:
# set target precision and confidence level
epsilon = 0.01
alpha = 0.05
problem = EstimationProblem(state_preparation=state_preparation, objective_qubits=[len(qr_state)])
# construct amplitude estimation
ae_cdf = IterativeAmplitudeEstimation(
epsilon_target=epsilon, alpha=alpha, sampler=Sampler(run_options={"shots": 100, "seed": 75})
)
result_cdf = ae_cdf.estimate(problem)
# print results
conf_int = np.array(result_cdf.confidence_interval)
print("Exact value: \t%.4f" % cdf[x_eval])
print("Estimated value:\t%.4f" % result_cdf.estimation)
print("Confidence interval: \t[%.4f, %.4f]" % tuple(conf_int))
Exact value: 0.9521
Estimated value: 0.9590
Confidence interval: [0.9577, 0.9603]
ঝুঁকিপূর্ণ মান#
নিম্নলিখিতটিতে আমরা ঝুঁকির মূল্য নির্ধারণের জন্য CDF কে দক্ষতার সাথে মূল্যায়নের জন্য দ্বিখণ্ডিত অনুসন্ধান (bisection search) এবং QAE ব্যবহার করি।
[23]:
def run_ae_for_cdf(x_eval, epsilon=0.01, alpha=0.05):
# construct amplitude estimation
state_preparation = get_cdf_circuit(x_eval)
problem = EstimationProblem(
state_preparation=state_preparation, objective_qubits=[len(qr_state)]
)
ae_var = IterativeAmplitudeEstimation(
epsilon_target=epsilon, alpha=alpha, sampler=Sampler(run_options={"shots": 100, "seed": 75})
)
result_var = ae_var.estimate(problem)
return result_var.estimation
[24]:
def bisection_search(
objective, target_value, low_level, high_level, low_value=None, high_value=None
):
"""
Determines the smallest level such that the objective value is still larger than the target
:param objective: objective function
:param target: target value
:param low_level: lowest level to be considered
:param high_level: highest level to be considered
:param low_value: value of lowest level (will be evaluated if set to None)
:param high_value: value of highest level (will be evaluated if set to None)
:return: dictionary with level, value, num_eval
"""
# check whether low and high values are given and evaluated them otherwise
print("--------------------------------------------------------------------")
print("start bisection search for target value %.3f" % target_value)
print("--------------------------------------------------------------------")
num_eval = 0
if low_value is None:
low_value = objective(low_level)
num_eval += 1
if high_value is None:
high_value = objective(high_level)
num_eval += 1
# check if low_value already satisfies the condition
if low_value > target_value:
return {
"level": low_level,
"value": low_value,
"num_eval": num_eval,
"comment": "returned low value",
}
elif low_value == target_value:
return {"level": low_level, "value": low_value, "num_eval": num_eval, "comment": "success"}
# check if high_value is above target
if high_value < target_value:
return {
"level": high_level,
"value": high_value,
"num_eval": num_eval,
"comment": "returned low value",
}
elif high_value == target_value:
return {
"level": high_level,
"value": high_value,
"num_eval": num_eval,
"comment": "success",
}
# perform bisection search until
print("low_level low_value level value high_level high_value")
print("--------------------------------------------------------------------")
while high_level - low_level > 1:
level = int(np.round((high_level + low_level) / 2.0))
num_eval += 1
value = objective(level)
print(
"%2d %.3f %2d %.3f %2d %.3f"
% (low_level, low_value, level, value, high_level, high_value)
)
if value >= target_value:
high_level = level
high_value = value
else:
low_level = level
low_value = value
# return high value after bisection search
print("--------------------------------------------------------------------")
print("finished bisection search")
print("--------------------------------------------------------------------")
return {"level": high_level, "value": high_value, "num_eval": num_eval, "comment": "success"}
[25]:
# run bisection search to determine VaR
objective = lambda x: run_ae_for_cdf(x)
bisection_result = bisection_search(
objective, 1 - alpha, min(losses) - 1, max(losses), low_value=0, high_value=1
)
var = bisection_result["level"]
--------------------------------------------------------------------
start bisection search for target value 0.950
--------------------------------------------------------------------
low_level low_value level value high_level high_value
--------------------------------------------------------------------
-1 0.000 1 0.752 3 1.000
1 0.752 2 0.959 3 1.000
--------------------------------------------------------------------
finished bisection search
--------------------------------------------------------------------
[26]:
print("Estimated Value at Risk: %2d" % var)
print("Exact Value at Risk: %2d" % exact_var)
print("Estimated Probability: %.3f" % bisection_result["value"])
print("Exact Probability: %.3f" % cdf[exact_var])
Estimated Value at Risk: 2
Exact Value at Risk: 2
Estimated Probability: 0.959
Exact Probability: 0.952
শর্তাধীন ঝুঁকিপূর্ণ মান#
Last, we compute the CVaR, i.e. the expected value of the loss conditional to it being larger than or equal to the VaR. To do so, we evaluate a piecewise linear objective function
To normalize, we have to divide the resulting expected value by the VaR-probability, i.e.
[27]:
# define linear objective
breakpoints = [0, var]
slopes = [0, 1]
offsets = [0, 0] # subtract VaR and add it later to the estimate
f_min = 0
f_max = 3 - var
c_approx = 0.25
cvar_objective = LinearAmplitudeFunction(
agg.num_sum_qubits,
slopes,
offsets,
domain=(0, 2**agg.num_sum_qubits - 1),
image=(f_min, f_max),
rescaling_factor=c_approx,
breakpoints=breakpoints,
)
cvar_objective.draw()
[27]:
┌────┐ q158_0: ┤0 ├ │ │ q158_1: ┤1 ├ │ │ q159: ┤2 F ├ │ │ a83_0: ┤3 ├ │ │ a83_1: ┤4 ├ └────┘
[28]:
# define the registers for convenience and readability
qr_state = QuantumRegister(u.num_qubits, "state")
qr_sum = QuantumRegister(agg.num_sum_qubits, "sum")
qr_carry = QuantumRegister(agg.num_carry_qubits, "carry")
qr_obj = QuantumRegister(1, "objective")
qr_work = QuantumRegister(cvar_objective.num_ancillas - len(qr_carry), "work")
# define the circuit
state_preparation = QuantumCircuit(qr_state, qr_obj, qr_sum, qr_carry, qr_work, name="A")
# load the random variable
state_preparation.append(u, qr_state)
# aggregate
state_preparation.append(agg, qr_state[:] + qr_sum[:] + qr_carry[:])
# linear objective function
state_preparation.append(cvar_objective, qr_sum[:] + qr_obj[:] + qr_carry[:] + qr_work[:])
# uncompute aggregation
state_preparation.append(agg.inverse(), qr_state[:] + qr_sum[:] + qr_carry[:])
[28]:
<qiskit.circuit.instructionset.InstructionSet at 0x7fdfa88db7f0>
আবার আমরা প্রথমে কোয়ান্টাম সিমুলেশন ব্যবহার করব কোয়ান্টাম বর্তনী যাচাইয়ের জন্য।
[29]:
state_preparation_measure = state_preparation.measure_all(inplace=False)
sampler = Sampler()
job = sampler.run(state_preparation_measure)
binary_probabilities = job.result().quasi_dists[0].binary_probabilities()
[30]:
# evaluate the result
value = 0
for i, prob in binary_probabilities.items():
if prob > 1e-6 and i[-(len(qr_state) + 1)] == "1":
value += prob
# normalize and add VaR to estimate
value = cvar_objective.post_processing(value)
d = 1.0 - bisection_result["value"]
v = value / d if d != 0 else 0
normalized_value = v + var
print("Estimated CVaR: %.4f" % normalized_value)
print("Exact CVaR: %.4f" % exact_cvar)
Estimated CVaR: 3.5799
Exact CVaR: 3.0000
এরপর আমরা QAE চালিয়ে CVaR অনুমান করি।
[31]:
# set target precision and confidence level
epsilon = 0.01
alpha = 0.05
problem = EstimationProblem(
state_preparation=state_preparation,
objective_qubits=[len(qr_state)],
post_processing=cvar_objective.post_processing,
)
# construct amplitude estimation
ae_cvar = IterativeAmplitudeEstimation(
epsilon_target=epsilon, alpha=alpha, sampler=Sampler(run_options={"shots": 100, "seed": 75})
)
result_cvar = ae_cvar.estimate(problem)
[32]:
# print results
d = 1.0 - bisection_result["value"]
v = result_cvar.estimation_processed / d if d != 0 else 0
print("Exact CVaR: \t%.4f" % exact_cvar)
print("Estimated CVaR:\t%.4f" % (v + var))
Exact CVaR: 3.0000
Estimated CVaR: 3.3316
[33]:
import qiskit.tools.jupyter
%qiskit_version_table
%qiskit_copyright
Version Information
Software | Version |
---|---|
qiskit | None |
qiskit-terra | 0.45.0.dev0+c626be7 |
qiskit_ibm_provider | 0.6.1 |
qiskit_aer | 0.12.0 |
qiskit_algorithms | 0.2.0 |
qiskit_finance | 0.4.0 |
System information | |
Python version | 3.9.7 |
Python compiler | GCC 7.5.0 |
Python build | default, Sep 16 2021 13:09:58 |
OS | Linux |
CPUs | 2 |
Memory (Gb) | 5.778430938720703 |
Fri Aug 18 16:24:38 2023 EDT |
This code is a part of Qiskit
© Copyright IBM 2017, 2023.
This code is licensed under the Apache License, Version 2.0. You may
obtain a copy of this license in the LICENSE.txt file in the root directory
of this source tree or at http://www.apache.org/licenses/LICENSE-2.0.
Any modifications or derivative works of this code must retain this
copyright notice, and modified files need to carry a notice indicating
that they have been altered from the originals.
[ ]: