Quantum State Tomography¶
Quantum tomography is an experimental procedure to reconstruct a description of part of a quantum system from the measurement outcomes of a specific set of experiments. In particular, quantum state tomography reconstructs the density matrix of a quantum state by preparing the state many times and measuring them in a tomographically complete basis of measurement operators.
Note
This tutorial requires the qiskit-aer and qiskit-ibm-runtime
packages to run simulations. You can install them with python -m pip
install qiskit-aer qiskit-ibm-runtime
.
We first initialize a simulator to run the experiments on.
from qiskit_aer import AerSimulator
from qiskit_ibm_runtime.fake_provider import FakePerth
backend = AerSimulator.from_backend(FakePerth())
To run a state tomography experiment, we initialize the experiment with a circuit to
prepare the state to be measured. We can also pass in an
Operator
or a Statevector
to describe the preparation circuit.
import qiskit
from qiskit_experiments.framework import ParallelExperiment
from qiskit_experiments.library import StateTomography
# GHZ State preparation circuit
nq = 2
qc_ghz = qiskit.QuantumCircuit(nq)
qc_ghz.h(0)
qc_ghz.s(0)
for i in range(1, nq):
qc_ghz.cx(0, i)
# QST Experiment
qstexp1 = StateTomography(qc_ghz)
qstdata1 = qstexp1.run(backend, seed_simulation=100).block_for_results()
# Print results
display(qstdata1.analysis_results(dataframe=True))
name | experiment | components | value | quality | backend | run_time | trace | eigvals | raw_eigvals | rescaled_psd | fitter_metadata | conditional_probability | positive | |
---|---|---|---|---|---|---|---|---|---|---|---|---|---|---|
a8dc6dee | state | StateTomography | [Q0, Q1] | DensityMatrix([[ 0.46500651+0.j , -0.01... | None | aer_simulator_from(fake_perth) | None | 1.0 | [0.9118390623289906, 0.051634993656863096, 0.0... | [0.9118390623289906, 0.051634993656863096, 0.0... | False | {'fitter': 'linear_inversion', 'fitter_time': ... | 1.0 | True |
783c8836 | state_fidelity | StateTomography | [Q0, Q1] | 0.910645 | None | aer_simulator_from(fake_perth) | None | None | None | None | None | None | None | None |
8bb4d093 | positive | StateTomography | [Q0, Q1] | True | None | aer_simulator_from(fake_perth) | None | None | None | None | None | None | None | None |
Tomography Results¶
The main result for tomography is the fitted state, which is stored as a
DensityMatrix
object:
state_result = qstdata1.analysis_results("state", dataframe=True).iloc[0]
print(state_result.value)
DensityMatrix([[ 0.46500651+0.j , -0.01204427+0.00748698j,
0.00227865-0.01123047j, 0.0078125 -0.44140625j],
[-0.01204427-0.00748698j, 0.02360026+0.j ,
-0.01367188+0.00390625j, -0.01822917+0.00634766j],
[ 0.00227865+0.01123047j, -0.01367188-0.00390625j,
0.03792318+0.j , 0.01822917+0.00846354j],
[ 0.0078125 +0.44140625j, -0.01822917-0.00634766j,
0.01822917-0.00846354j, 0.47347005+0.j ]],
dims=(2, 2))
We can also visualize the density matrix:
from qiskit.visualization import plot_state_city
state = qstdata1.analysis_results("state", dataframe=True).iloc[0].value
plot_state_city(state, title='Density Matrix')
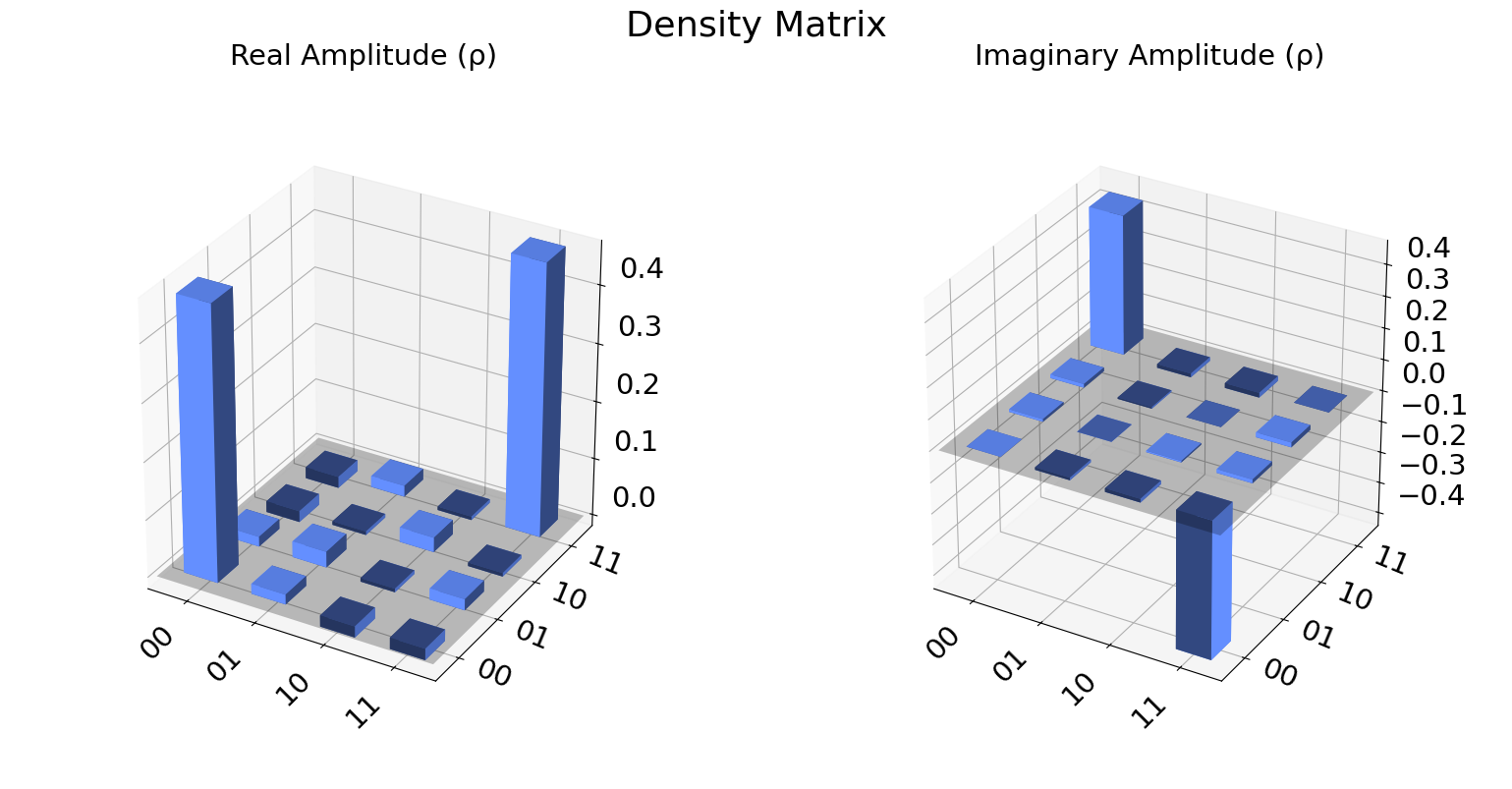
The state fidelity of the fitted state with the ideal state prepared by
the input circuit is stored in the "state_fidelity"
result field.
Note that if the input circuit contained any measurements the ideal
state cannot be automatically generated and this field will be set to
None
.
fid_result = qstdata1.analysis_results("state_fidelity", dataframe=True).iloc[0]
print("State Fidelity = {:.5f}".format(fid_result.value))
State Fidelity = 0.91064
Additional state metadata¶
Additional data is stored in the tomography under additional fields. This includes
eigvals
: the eigenvalues of the fitted statetrace
: the trace of the fitted statepositive
: Whether the eigenvalues are all non-negative
If trace rescaling was performed this dictionary will also contain a raw_trace
field
containing the trace before rescaling. Futhermore, if the state was rescaled to be
positive or trace 1 an additional field raw_eigvals
will contain the state
eigenvalues before rescaling was performed.
for col in ["eigvals", "trace", "positive"]:
print(f"{col}: {state_result[col]}")
eigvals: [0.91183906 0.05163499 0.02271696 0.01380898]
trace: 1.0000000000000013
positive: True
To see the effect of rescaling, we can perform a “bad” fit with very low counts:
# QST Experiment
bad_data = qstexp1.run(backend, shots=10, seed_simulation=100).block_for_results()
bad_state_result = bad_data.analysis_results("state", dataframe=True).iloc[0]
# Print result
for key, val in bad_state_result.items():
print(f"{key}: {val}")
name: state
experiment: StateTomography
components: [<Qubit(Q0)>, <Qubit(Q1)>]
value: DensityMatrix([[ 0.35133904+0.00000000e+00j, -0.05460501-1.30768269e-02j,
-0.0296055 +1.47087084e-01j, 0.11114873-3.27070794e-01j],
[-0.05460501+1.30768269e-02j, 0.09319463-3.46944695e-18j,
0.03814685-5.05548020e-02j, 0.06972563+4.37987876e-02j],
[-0.0296055 -1.47087084e-01j, 0.03814685+5.05548020e-02j,
0.10005139+1.73472348e-18j, -0.1102921 -2.17247025e-02j],
[ 0.11114873+3.27070794e-01j, 0.06972563-4.37987876e-02j,
-0.1102921 +2.17247025e-02j, 0.45541494+2.77555756e-17j]],
dims=(2, 2))
quality: None
backend: aer_simulator_from(fake_perth)
run_time: None
trace: 1.000000000000006
eigvals: [0.81115115 0.1671573 0.02169155 0. ]
raw_eigvals: [ 0.88335141 0.23935756 0.09389182 -0.21660079]
rescaled_psd: True
fitter_metadata: {'fitter': 'linear_inversion', 'fitter_time': 0.004080772399902344}
conditional_probability: 1.0
positive: True
Tomography Fitters¶
The default fitters is linear_inversion
, which reconstructs the
state using dual basis of the tomography basis. This will typically
result in a non-positive reconstructed state. This state is rescaled to
be positive-semidefinite (PSD) by computing its eigen-decomposition and
rescaling its eigenvalues using the approach from Ref. [1].
There are several other fitters are included (See API documentation for
details). For example, if cvxpy
is installed we can use the
cvxpy_gaussian_lstsq()
fitter, which allows constraining the fit to be
PSD without requiring rescaling.
try:
import cvxpy
# Set analysis option for cvxpy fitter
qstexp1.analysis.set_options(fitter='cvxpy_gaussian_lstsq')
# Re-run experiment
qstdata2 = qstexp1.run(backend, seed_simulation=100).block_for_results()
state_result2 = qstdata2.analysis_results("state", dataframe=True).iloc[0]
for key, val in state_result2.items():
print(f"{key}: {val}")
except ModuleNotFoundError:
print("CVXPY is not installed")
name: state
experiment: StateTomography
components: [<Qubit(Q0)>, <Qubit(Q1)>]
value: DensityMatrix([[ 4.58614058e-01+0.j , 5.98255698e-03+0.00125795j,
6.42806612e-05+0.00897406j, 5.12200875e-03-0.43504751j],
[ 5.98255698e-03-0.00125795j, 2.70696280e-02+0.j ,
-2.08977388e-03+0.00247464j, 2.05446931e-04+0.00176623j],
[ 6.42806612e-05-0.00897406j, -2.08977388e-03-0.00247464j,
4.29273330e-02+0.j , -1.34110440e-02+0.00402144j],
[ 5.12200875e-03+0.43504751j, 2.05446931e-04-0.00176623j,
-1.34110440e-02-0.00402144j, 4.71388981e-01+0.j ]],
dims=(2, 2))
quality: None
backend: aer_simulator_from(fake_perth)
run_time: None
trace: 0.9999999898537167
eigvals: [0.90043866 0.04404499 0.03406292 0.02145343]
raw_eigvals: [0.90043867 0.04404499 0.03406292 0.02145343]
rescaled_psd: False
fitter_metadata: {'fitter': 'cvxpy_gaussian_lstsq', 'cvxpy_solver': 'SCS', 'cvxpy_status': ['optimal'], 'psd_constraint': True, 'trace_preserving': True, 'fitter_time': 0.032004356384277344}
conditional_probability: 1.0
positive: True
Parallel Tomography Experiment¶
We can also use the ParallelExperiment
class to
run subsystem tomography on multiple qubits in parallel.
For example if we want to perform 1-qubit QST on several qubits at once:
from math import pi
num_qubits = 5
gates = [qiskit.circuit.library.RXGate(i * pi / (num_qubits - 1))
for i in range(num_qubits)]
subexps = [
StateTomography(gate, physical_qubits=(i,))
for i, gate in enumerate(gates)
]
parexp = ParallelExperiment(subexps)
pardata = parexp.run(backend, seed_simulation=100).block_for_results()
display(pardata.analysis_results(dataframe=True))
name | experiment | components | value | quality | backend | run_time | trace | eigvals | raw_eigvals | rescaled_psd | fitter_metadata | conditional_probability | positive | |
---|---|---|---|---|---|---|---|---|---|---|---|---|---|---|
acd5171b | state | StateTomography | [Q0] | DensityMatrix([[0.96972656+0.j , 0. ... | None | aer_simulator_from(fake_perth) | None | 1.0 | [0.9700553520560977, 0.029944647943903127] | [0.9700553520560977, 0.029944647943903127] | False | {'fitter': 'linear_inversion', 'fitter_time': ... | 1.0 | True |
e5198d37 | state_fidelity | StateTomography | [Q0] | 0.969727 | None | aer_simulator_from(fake_perth) | None | None | None | None | None | None | None | None |
2c05c5b0 | positive | StateTomography | [Q0] | True | None | aer_simulator_from(fake_perth) | None | None | None | None | None | None | None | None |
e21d9bbe | state | StateTomography | [Q1] | DensityMatrix([[0.86328125+0.j , 0.0214... | None | aer_simulator_from(fake_perth) | None | 1.0 | [0.999259400242468, 0.000740599757532906] | [0.999259400242468, 0.000740599757532906] | False | {'fitter': 'linear_inversion', 'fitter_time': ... | 1.0 | True |
081d1f15 | state_fidelity | StateTomography | [Q1] | 0.998566 | None | aer_simulator_from(fake_perth) | None | None | None | None | None | None | None | None |
05dd38d8 | positive | StateTomography | [Q1] | True | None | aer_simulator_from(fake_perth) | None | None | None | None | None | None | None | None |
1a13c39f | state | StateTomography | [Q2] | DensityMatrix([[ 0.49023438+0.j , -0.018... | None | aer_simulator_from(fake_perth) | None | 1.0 | [0.9614141553594923, 0.038585844640508626] | [0.9614141553594923, 0.038585844640508626] | False | {'fitter': 'linear_inversion', 'fitter_time': ... | 1.0 | True |
916698fc | state_fidelity | StateTomography | [Q2] | 0.960938 | None | aer_simulator_from(fake_perth) | None | None | None | None | None | None | None | None |
a7f3d3f2 | positive | StateTomography | [Q2] | True | None | aer_simulator_from(fake_perth) | None | None | None | None | None | None | None | None |
6864b2e6 | state | StateTomography | [Q3] | DensityMatrix([[0.16503906+0.j , 0.0117... | None | aer_simulator_from(fake_perth) | None | 1.0 | [0.9738512302435445, 0.026148769756456525] | [0.9738512302435445, 0.026148769756456525] | False | {'fitter': 'linear_inversion', 'fitter_time': ... | 1.0 | True |
920a299b | state_fidelity | StateTomography | [Q3] | 0.973706 | None | aer_simulator_from(fake_perth) | None | None | None | None | None | None | None | None |
70fc0549 | positive | StateTomography | [Q3] | True | None | aer_simulator_from(fake_perth) | None | None | None | None | None | None | None | None |
48056f55 | state | StateTomography | [Q4] | DensityMatrix([[ 0.02734375+0.j , -0.0156... | None | aer_simulator_from(fake_perth) | None | 1.0 | [0.9731724969966684, 0.02682750300333219] | [0.9731724969966684, 0.02682750300333219] | False | {'fitter': 'linear_inversion', 'fitter_time': ... | 1.0 | True |
f826e349 | state_fidelity | StateTomography | [Q4] | 0.972656 | None | aer_simulator_from(fake_perth) | None | None | None | None | None | None | None | None |
795cca59 | positive | StateTomography | [Q4] | True | None | aer_simulator_from(fake_perth) | None | None | None | None | None | None | None | None |
View experiment analysis results for one component:
results = pardata.analysis_results(dataframe=True)
display(results[results.components.apply(lambda x: x == ["Q0"])])
name | experiment | components | value | quality | backend | run_time | trace | eigvals | raw_eigvals | rescaled_psd | fitter_metadata | conditional_probability | positive | |
---|---|---|---|---|---|---|---|---|---|---|---|---|---|---|
acd5171b | state | StateTomography | [Q0] | DensityMatrix([[0.96972656+0.j , 0. ... | None | aer_simulator_from(fake_perth) | None | 1.0 | [0.9700553520560977, 0.029944647943903127] | [0.9700553520560977, 0.029944647943903127] | False | {'fitter': 'linear_inversion', 'fitter_time': ... | 1.0 | True |
e5198d37 | state_fidelity | StateTomography | [Q0] | 0.969727 | None | aer_simulator_from(fake_perth) | None | None | None | None | None | None | None | None |
2c05c5b0 | positive | StateTomography | [Q0] | True | None | aer_simulator_from(fake_perth) | None | None | None | None | None | None | None | None |
References¶
See also¶
API documentation:
StateTomography