Note
This is the documentation for the current state of the development branch of Qiskit Experiments. The documentation or APIs here can change prior to being released.
T1 Characterization¶
Background¶
In a
We start by fixing a delay time
In the absence of state preparation and measurement errors, the
probability to measure
The following code demonstrates a basic run of a
Note
This tutorial requires the qiskit-aer and qiskit-ibm-runtime
packages to run simulations. You can install them with python -m pip
install qiskit-aer qiskit-ibm-runtime
.
import numpy as np
from qiskit_experiments.framework import MeasLevel, ParallelExperiment
from qiskit_experiments.library import T1
from qiskit_experiments.library.characterization.analysis.t1_analysis import T1KerneledAnalysis
# A T1 simulator
from qiskit_aer import AerSimulator
from qiskit_aer.noise import NoiseModel
from qiskit_ibm_runtime.fake_provider import FakePerth
# A kerneled data simulator
from qiskit_experiments.test.mock_iq_backend import MockIQBackend
from qiskit_experiments.test.mock_iq_helpers import MockIQT1Helper
# Create a pure relaxation noise model for AerSimulator
noise_model = NoiseModel.from_backend(
FakePerth(), thermal_relaxation=True, gate_error=False, readout_error=False
)
# Create a fake backend simulator
backend = AerSimulator.from_backend(FakePerth(), noise_model=noise_model)
# Look up target T1 of qubit-0 from device properties
qubit0_t1 = FakePerth().qubit_properties(0).t1
# Time intervals to wait before measurement
delays = np.arange(1e-6, 3 * qubit0_t1, 3e-5)
# Create an experiment for qubit 0
# with the specified time intervals
exp = T1(physical_qubits=(0,), delays=delays)
# Set scheduling method so circuit is scheduled for delay noise simulation
exp.set_transpile_options(scheduling_method='asap')
# Run the experiment circuits and analyze the result
exp_data = exp.run(backend=backend, seed_simulator=101).block_for_results()
# Print the result
display(exp_data.figure(0))
print(exp_data.analysis_results(dataframe=True))
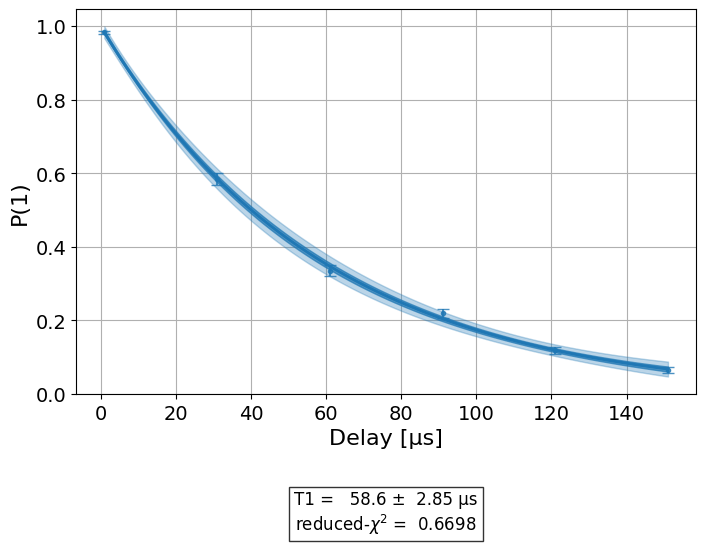
name experiment components value quality \
18ee9e4f T1 T1 [Q0] (5.86+/-0.28)e-05 good
backend run_time chisq unit
18ee9e4f aer_simulator_from(fake_perth) None 0.669797 s
experiments with kerneled measurement¶
meas_level=MeasLevel.KERNELED
, the job
will not discriminate the IQ data and will not label it. In the T1 experiment,
since we know that
# Experiment
ns = 1e-9
mu = 1e-6
# qubit properties
t1 = 45 * mu
# we will guess that our guess is 10% off the exact value of t1 for qubit 0.
t1_estimated_shift = t1/10
# We use log space for the delays because of the noise properties
delays = np.logspace(1, 11, num=23, base=np.exp(1))
delays *= ns
# Adding circuits with delay=0 and long delays so the centers in the IQ plane won't be misplaced.
# Without this, the fitting can provide wrong results.
delays = np.insert(delays, 0, 0)
delays = np.append(delays, [t1*3])
num_qubits = 2
num_shots = 2048
backend = MockIQBackend(
MockIQT1Helper(
t1=t1,
iq_cluster_centers=[((-5.0, -4.0), (-5.0, 4.0)), ((3.0, 1.0), (5.0, -3.0))],
iq_cluster_width=[1.0, 2.0],
)
)
# Creating a T1 experiment
expT1_kerneled = T1((0,), delays)
expT1_kerneled.analysis = T1KerneledAnalysis()
expT1_kerneled.analysis.set_options(p0={"amp": 1, "tau": t1 + t1_estimated_shift, "base": 0})
# Running the experiment
expdataT1_kerneled = expT1_kerneled.run(
backend=backend, meas_return="avg", meas_level=MeasLevel.KERNELED, shots=num_shots
).block_for_results()
# Displaying results
display(expdataT1_kerneled.figure(0))
display(expdataT1_kerneled.analysis_results(dataframe=True))
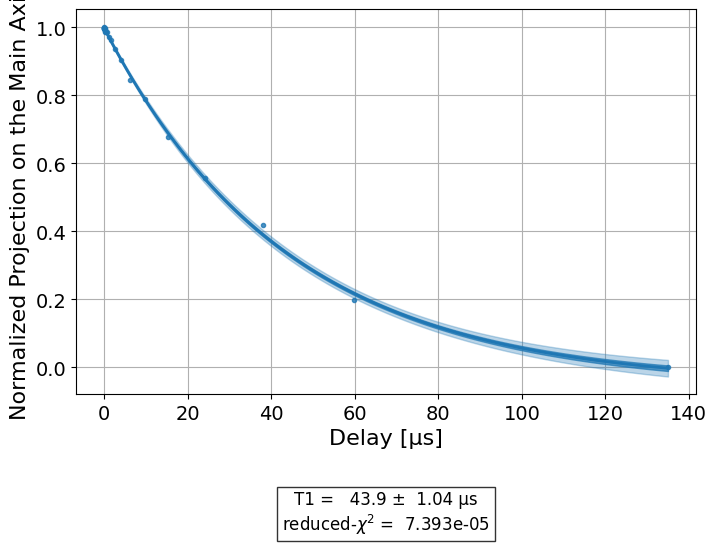
name | experiment | components | value | quality | backend | run_time | chisq | unit | |
---|---|---|---|---|---|---|---|---|---|
c683c90a | T1 | T1 | [Q0] | (4.39+/-0.10)e-05 | good | <qiskit_experiments.test.mock_iq_backend.MockI... | None | 0.000074 | s |
See also¶
API documentation:
T1